The first Genie Nano camera model with a quad-polarizer filter using the Sony Pregius IMX250-MZR 5.1MP monochrome image sensor is now available. The Teledyne Dalsa Nano M2450 camera incorporates the nanowire polarizer filter allowing detection of both the angle and amount of polarized light.
What problems can the Nano M2450 polarized camera solve?
Polarized filtering can reduce the effects of reflections and glare from multiple directions and reveal otherwise undetectable features in the target scene. Polarization enables detection of stress, birefringence, through-reflection and glare from surfaces like glass, plastic, and metal. Sony’s newest image sensor, with its pixel-level polarizer structure, enables the detection of both the amount and angle of polarized light across a scene.
Four different angled polarizers (90°, 45°, 135° and 0°) are positioned on each pixel, and every block of four pixels comprises a calculation unit.
How does polarization work? Theory of operation
Polarization direction is defined as the electrical direction. Light, with its electrical field oscillating perpendicular to the nano wire grid, passes through the filter while that in the parallel direction is rejected.
For Polarized light, only the portion of the light vector perpendicular to the angle of the nanowire filter grid passes.
For example, with a wire-grid polarizer filter at 90 deg. to the maximum transmission is for polarized light at an angle of 0 deg.
The polarizer filter is placed directly on the sensor’s pixel array, beneath the micro-lens array. This design, compared to polarize filters on top of the micro lens array reduced the possibility of light at a polarized angle being misdirected into adjacent pixels (cross talk) and incorrectly detected at the wrong angle.
The Genie Nano’s polarizer filter on the camera sensor is a 2 x 2 pattern, with each pixel having a nanowire polarizer filter with different angles (90, 45, 135 and 0 degree’s)
The image output pattern of the monochrome camera is arranged in 2 x 2 pixel block as follows:
That is, the first line output is an alternating sequence of pixels 0 & 35 degrees, with the following line of 45 and 90 degrees.
Given the proportion of light available through these four filters, any angle of polarized light can be calculated. Any given state of polarization can be composed by two linearly polarized waves in perpendicular directions. The state of polarization is determined by the relative amplitude and difference in phase between the two component waves.
Calculations on the 2×2 filter blocks result in a single pixel for each polarizer filter angle, therefore the resulting image is one fourth the original image resolution. For example, with an original image of 2464×2056, the resulting image is 1232×1028 (original buffer width/2 and original buffer height/2) for a single polarizing angle.
Teledyne Dalsa offers a Polarization demo user interface making it easy to test the polarization techniques for various applications. This includes the ability to see the results of various processing algorithms with the summed images.
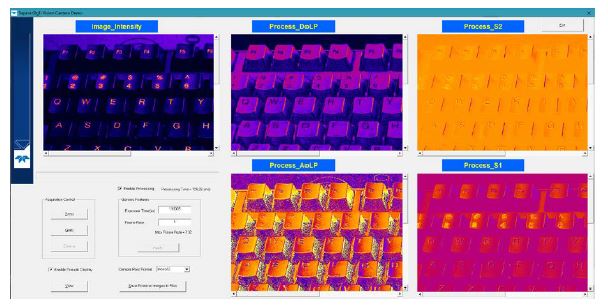
In summary, the new Dalsa Nano M2450 polarized camera can help resolve defects not detected by traditional imaging! Contact 1st Vision to arrange a camera demo in which we will provide the demo polarization software as well or discuss your application. Or click HERE to request a quote
Need line scan? – With the addition of the Genie Nano polarized model, Teledyne DALSA is the first company to offer polarization for both area and line scan (Piranha™4 polarization) cameras
1st Vision’s sales engineers have over 100 years of combined experience to assist in your camera selection. With a large portfolio of lenses, cables, NIC card and industrial computers, we can provide a full vision solution!
Related Posts
Dalsa line scan polarization camera makes invisible visible!
Teledyne Dalsa TurboDrive 2.0 breaks past GigE limits now with 6 levels of compression